Intro
Discover causal inference techniques, including regression, propensity scores, and instrumental variables, to establish cause-and-effect relationships and make data-driven decisions with confidence.
Causal inference techniques have become increasingly important in various fields, including economics, medicine, and social sciences. The ability to establish cause-and-effect relationships between variables is crucial for making informed decisions, predicting outcomes, and evaluating the effectiveness of interventions. In this article, we will delve into the world of causal inference, exploring its significance, key concepts, and various techniques used to establish causal relationships.
Causal inference is a critical aspect of scientific inquiry, as it enables researchers to identify the underlying mechanisms that drive phenomena. By understanding the causal relationships between variables, researchers can develop more accurate models, make predictions, and design effective interventions. The importance of causal inference cannot be overstated, as it has far-reaching implications for policy-making, business decision-making, and personal decision-making. For instance, in medicine, understanding the causal relationship between a treatment and a disease outcome is essential for developing effective treatments and improving patient care.
The concept of causal inference is rooted in the idea that correlation does not necessarily imply causation. In other words, just because two variables are related, it does not mean that one causes the other. To establish causality, researchers must use specialized techniques that account for confounding variables, selection bias, and other sources of error. These techniques are designed to help researchers identify the underlying causal mechanisms that drive the relationships between variables. Some of the key concepts in causal inference include confounding variables, instrumental variables, and causal graphs.
Causal Inference Techniques
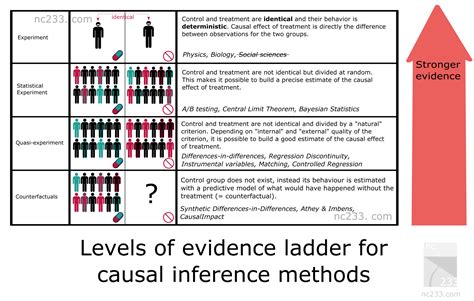
Causal inference techniques can be broadly classified into two categories: experimental and observational. Experimental techniques involve manipulating the variable of interest and measuring its effect on the outcome variable. Observational techniques, on the other hand, involve analyzing existing data to identify causal relationships. Some of the most commonly used causal inference techniques include regression analysis, propensity score matching, and instrumental variable analysis. Each of these techniques has its strengths and limitations, and the choice of technique depends on the research question, data quality, and study design.
Experimental Techniques
Experimental techniques are considered the gold standard for establishing causality. These techniques involve randomly assigning participants to treatment or control groups and measuring the effect of the treatment on the outcome variable. Randomized controlled trials (RCTs) are a type of experimental technique that is widely used in medicine and social sciences. RCTs are designed to minimize confounding variables and selection bias, allowing researchers to establish causality with a high degree of confidence. However, experimental techniques can be time-consuming, expensive, and may not be feasible in all situations.Observational Techniques
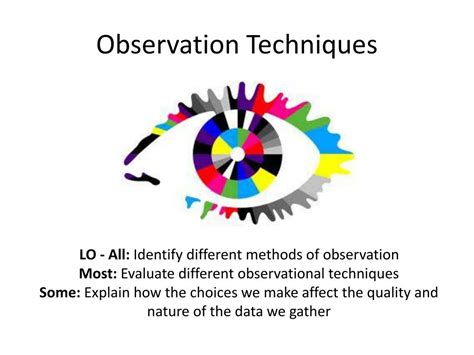
Observational techniques are used when experimental techniques are not feasible. These techniques involve analyzing existing data to identify causal relationships. Some of the most commonly used observational techniques include regression analysis, propensity score matching, and instrumental variable analysis. Regression analysis is a statistical technique that is used to model the relationship between a dependent variable and one or more independent variables. Propensity score matching is a technique that is used to match participants in the treatment and control groups based on their propensity scores. Instrumental variable analysis is a technique that is used to identify causal relationships by exploiting exogenous variation in the data.
Regression Analysis
Regression analysis is a statistical technique that is widely used in causal inference. The technique involves modeling the relationship between a dependent variable and one or more independent variables. The goal of regression analysis is to identify the relationship between the independent variables and the dependent variable, while controlling for confounding variables. There are several types of regression analysis, including linear regression, logistic regression, and generalized linear regression. Each of these types has its strengths and limitations, and the choice of technique depends on the research question and data quality.Propensity Score Matching
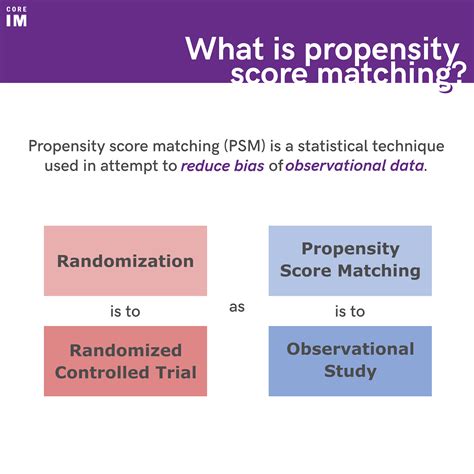
Propensity score matching is a technique that is used to match participants in the treatment and control groups based on their propensity scores. The propensity score is the probability of being assigned to the treatment group, given a set of observed characteristics. By matching participants based on their propensity scores, researchers can create a balanced sample that is similar to a randomized controlled trial. Propensity score matching is a powerful technique that can be used to establish causality in observational studies. However, the technique requires high-quality data and a robust model specification.
Instrumental Variable Analysis
Instrumental variable analysis is a technique that is used to identify causal relationships by exploiting exogenous variation in the data. The technique involves using an instrumental variable that is correlated with the treatment variable but not with the outcome variable. The instrumental variable is used to identify the causal effect of the treatment variable on the outcome variable. Instrumental variable analysis is a powerful technique that can be used to establish causality in observational studies. However, the technique requires a valid instrumental variable, which can be difficult to find.Causal Graphs
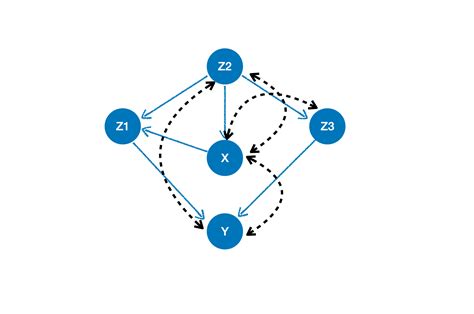
Causal graphs are a visual representation of the causal relationships between variables. The graphs are used to identify the underlying causal mechanisms that drive the relationships between variables. Causal graphs are a powerful tool that can be used to communicate complex causal relationships in a simple and intuitive way. The graphs are also useful for identifying potential sources of bias and confounding variables.
Confounding Variables
Confounding variables are variables that are related to both the treatment variable and the outcome variable. These variables can bias the estimate of the causal effect if they are not properly controlled for. Confounding variables can be controlled for using various techniques, including regression analysis, propensity score matching, and instrumental variable analysis. It is essential to identify and control for confounding variables to establish causality.Applications of Causal Inference
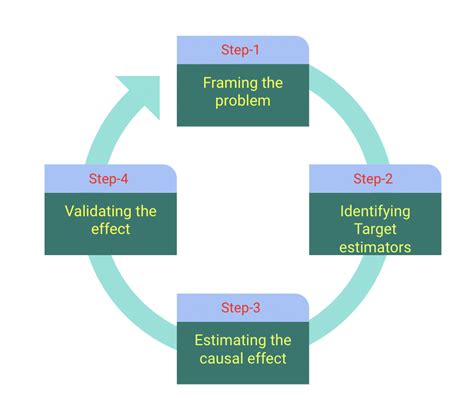
Causal inference has a wide range of applications in various fields, including economics, medicine, and social sciences. In economics, causal inference is used to evaluate the effectiveness of policies and programs. In medicine, causal inference is used to develop effective treatments and improve patient care. In social sciences, causal inference is used to understand the underlying mechanisms that drive social phenomena.
Economics
In economics, causal inference is used to evaluate the effectiveness of policies and programs. For example, researchers may use causal inference techniques to evaluate the impact of a tax policy on economic growth. The goal of the analysis is to identify the causal effect of the tax policy on economic growth, while controlling for confounding variables.Medicine
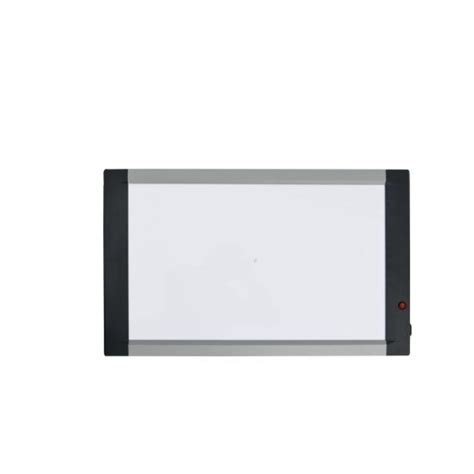
In medicine, causal inference is used to develop effective treatments and improve patient care. For example, researchers may use causal inference techniques to evaluate the effectiveness of a new drug. The goal of the analysis is to identify the causal effect of the drug on the outcome variable, while controlling for confounding variables.
Social Sciences
In social sciences, causal inference is used to understand the underlying mechanisms that drive social phenomena. For example, researchers may use causal inference techniques to evaluate the impact of education on income. The goal of the analysis is to identify the causal effect of education on income, while controlling for confounding variables.Causal Inference Image Gallery
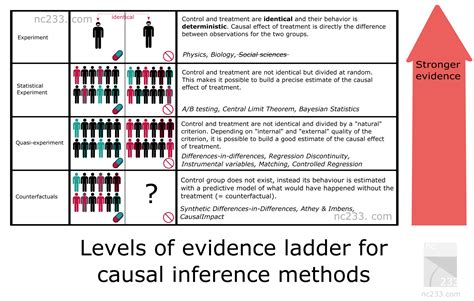
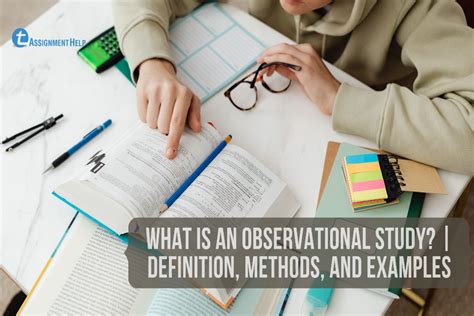
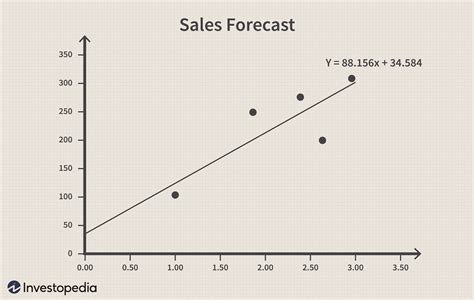
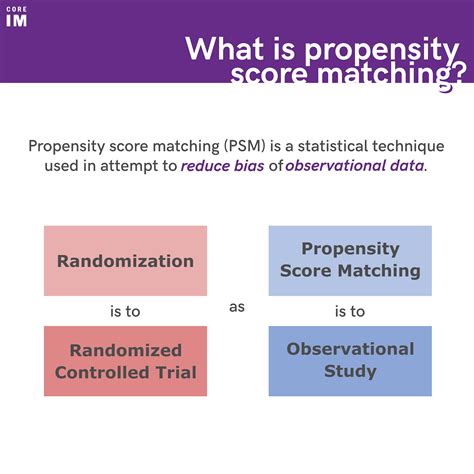
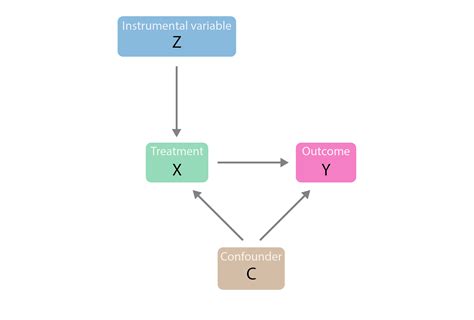
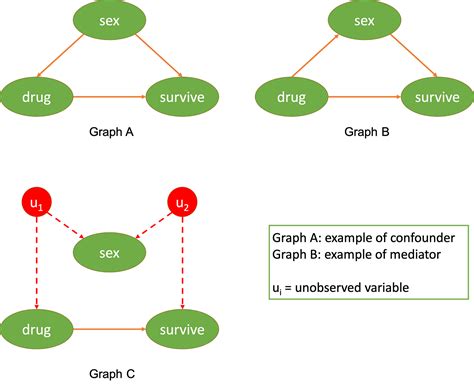
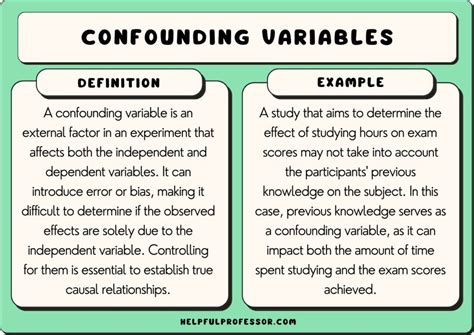
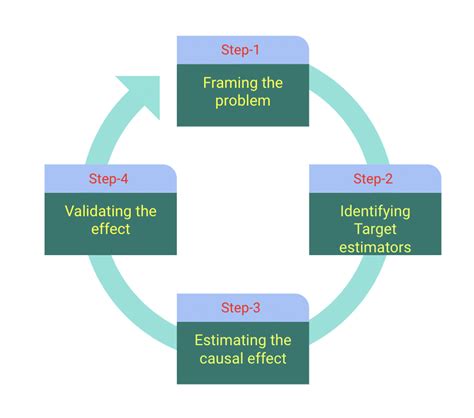
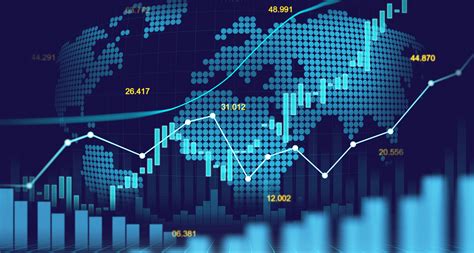
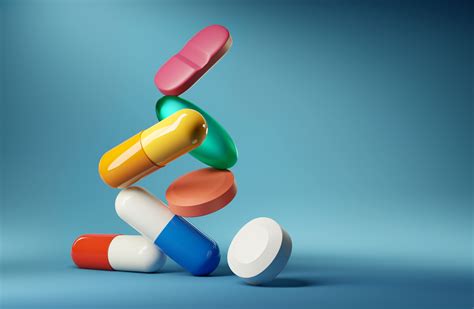
In conclusion, causal inference techniques are essential for establishing cause-and-effect relationships between variables. The techniques are widely used in various fields, including economics, medicine, and social sciences. By understanding the underlying causal mechanisms that drive phenomena, researchers can develop more accurate models, make predictions, and design effective interventions. We encourage readers to share their thoughts and experiences with causal inference techniques in the comments section below. Additionally, we invite readers to explore the applications of causal inference in their respective fields and to consider the potential benefits of using these techniques in their own research.